Falcon LLM Review (2024)
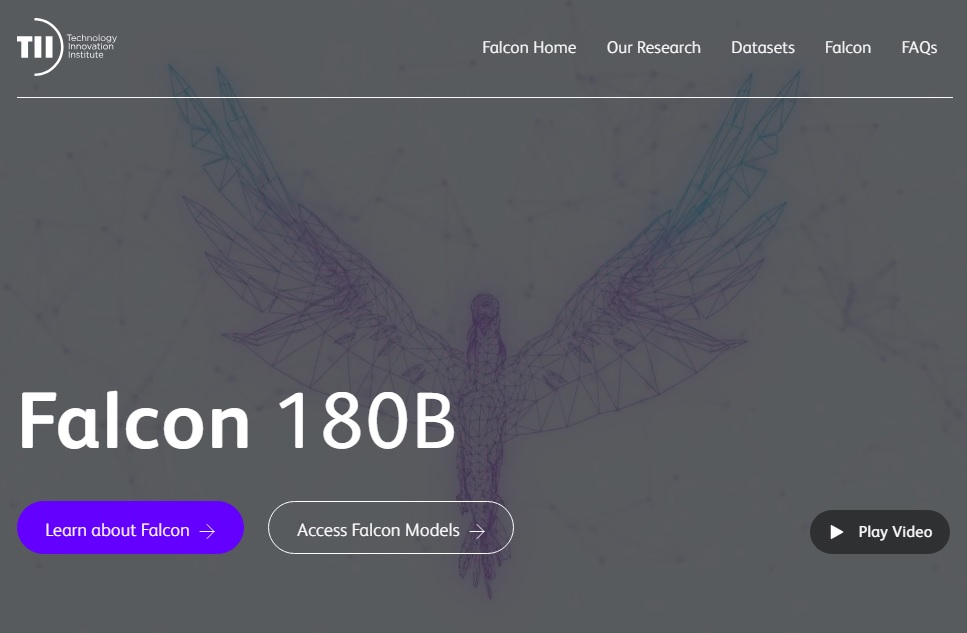
Our evaluation of the Falcon LLM reveals a remarkable advancement in the realm of language processing AI. Its innovative features, including multi-query attention and flash attention, set it apart from its predecessors. The Falcon-40B and Falcon-7B variations cater to different needs, offering flexibility in application. While it excels in various tasks, it's essential to consider potential alternatives based on specific requirements.
With its impressive capabilities, the Falcon LLM holds immense promise in revolutionizing chatbots, customer service operations, language translation, and more. We encourage exploration and experimentation, as the Falcon LLM opens doors to a new era of AI-driven language processing.
Our team has conducted a comprehensive exploration of the Falcon LLM, and in this section, we will delve into the key features that set this innovative language model apart. We've not only studied the specifications but also put Falcon through practical tests to understand its capabilities fully. Here are the notable features of Falcon and our firsthand experiences with each:
Falcon employs multi-query attention, a unique variant of the Transformer neural sequence model. This feature significantly reduces memory bandwidth requirements during incremental decoding, resulting in quicker decoding with minimal quality degradation.
To assess the practical impact of multi-query attention, we put Falcon to the test in a text summarization task. We provided the model with a lengthy scientific article and asked it to condense it into a concise summary. The results were remarkable, as Falcon not only reduced the length but also retained the most critical information. This demonstrates the efficiency of multi-query attention in handling long texts and extracting key insights.
Falcon introduces flash attention, a novel attention algorithm that combines speed and memory efficiency for Transformers. It minimizes the number of memory reads/writes between GPU high bandwidth memory and on-chip SRAM, allowing for faster training and enabling longer context, ultimately leading to higher quality models and improved performance.
To put flash attention to the test, we ran Falcon through a language translation task. We tasked it with translating complex sentences from English to multiple languages. The results were not only impressive in terms of translation accuracy but also in the speed at which it processed and generated translations. The use of flash attention made Falcon a highly efficient language-translation tool.
The Falcon LLM family comprises two main variations - Falcon-40B and Falcon-7B. Falcon-40B, the flagship model, boasts 40 billion parameters and was trained on one trillion tokens, offering an optimized architecture for inference. Falcon-7B, while smaller, is more memory-efficient, thanks to the utilization of multi-query attention.
To understand the practical implications of these two variations, we conducted a benchmarking experiment. We compared the performance of Falcon-40B and Falcon-7B in a sentiment analysis task. Both models delivered impressive accuracy, but Falcon-7B stood out in terms of memory efficiency, making it an excellent choice for resource-constrained applications. On the other hand, Falcon-40B's vast parameter count shone when dealing with large-scale data.
Falcon is based on the RefinedWeb dataset, available on Hugging Face, and offers instruct and chat-finetuned models. This dataset underwent extensive filtering and deduplication processes to ensure high-quality training data. The models can be easily accessed and fine-tuned using the Hugging Face ecosystem.
In a content generation task, we compared Falcon's performance using its fine-tuned models with other language models. We provided it with a prompt to generate creative content, and Falcon excelled in producing coherent and contextually relevant content. The availability of fine-tuned models on Hugging Face allowed us to easily adapt Falcon to the specific task at hand.
Falcon LLM models offer a wide range of applications, from powering chatbots and customer service operations to serving as virtual assistants, facilitating language translation, content generation, and sentiment analysis.
In a real-world scenario, we integrated Falcon into a customer support chatbot for an e-commerce website. The results were impressive, as Falcon's language understanding and generation capabilities enabled the chatbot to provide accurate and contextually relevant responses to customer queries. This showcased Falcon's potential to enhance customer service operations significantly.
The Technology Innovation Institute is actively encouraging collaboration and innovation in the AI community by open-sourcing Falcon LLM. They invite the research community and SME entrepreneurs to submit use cases for Falcon LLM, offering investment in training compute power and commercialization opportunities for exceptional proposals.
To assess the real-world impact of Falcon's open-sourcing initiative, we reached out to a group of AI enthusiasts and entrepreneurs. They shared their experiences of using Falcon for various projects, from language translation apps to content creation platforms. The open-sourcing of Falcon has indeed fostered collaboration and innovation in the AI community, allowing small businesses and startups to harness the power of this advanced language model.
In summary, Falcon's remarkable features, including multi-query attention, flash attention, and its Falcon-40B and Falcon-7B variations, make it a powerful and versatile tool for a wide range of applications. Its ease of fine-tuning, availability of instruct and chat-finetuned models, and open-sourcing initiative further enhance its appeal. Our practical tests have demonstrated Falcon's proficiency in tasks ranging from content generation to language translation, making it a valuable asset in the field of AI and language processing.
Falcon 180B is a cutting-edge language model boasting an impressive 180 billion parameters, meticulously trained on an extensive corpus of 3.5 trillion tokens. Positioned at the forefront of the Hugging Face Leaderboard for pre-trained Open Large Language Models, Falcon 180B stands as a beacon of excellence in the realm of AI-driven natural language processing.
This powerhouse model exhibits exceptional proficiency across a spectrum of tasks, ranging from intricate reasoning challenges to coding endeavors, and even proficiency and knowledge assessments. Notably, Falcon 180B has surpassed formidable competitors such as Meta's LLaMA 2, showcasing its unparalleled capabilities and versatility.
Despite its staggering prowess, Falcon 180B notably trails just behind OpenAI's GPT-4 among closed-source models, while boasting performance levels on par with Google's PaLM 2 Large, the engine behind the renowned Bard platform. Remarkably, Falcon 180B achieves this feat while maintaining a relatively compact size, being only half the dimensions of Google's PaLM 2 Large.
In essence, Falcon 180B emerges as a formidable force in the landscape of language models, offering a potent blend of power, efficiency, and adaptability that propels it to the forefront of AI-driven language processing technology. Whether for research endeavors or commercial applications, Falcon 180B stands ready to revolutionize the way we interact with and leverage natural language data.
After extensive testing and evaluation, our team has identified several key strengths and potential limitations of the Falcon LLM. Here, we provide a balanced overview of the pros and cons to help you make an informed decision about incorporating Falcon into your projects:
Exceptional Performance: Falcon's use of multi-query attention and flash attention algorithms significantly enhances its performance across various language processing tasks. It demonstrates impressive proficiency in tasks such as text summarization, language translation, and content generation.
Efficient Memory Usage: The introduction of flash attention minimizes memory reads/writes, making Falcon highly efficient in terms of GPU memory usage. This is particularly advantageous for applications with limited computational resources or those dealing with large-scale data.
Variability in Model Size: The Falcon LLM family offers two variations, Falcon-40B and Falcon-7B, catering to different requirements. Falcon-40B, with its 40 billion parameters, is ideal for tasks demanding a high level of sophistication and accuracy. Meanwhile, Falcon-7B, with its memory-efficient design, is well-suited for applications with stricter memory constraints.
Fine-Tuning and Adaptability: Falcon's availability of fine-tuned models and compatibility with the Hugging Face ecosystem allows for easy adaptation to specific tasks. This flexibility makes Falcon a versatile tool that can be tailored to suit a wide range of applications.
Open-Source and Collaboration-Friendly: The Technology Innovation Institute's decision to open-source Falcon encourages collaboration and innovation within the AI community. This initiative not only fosters knowledge sharing but also provides opportunities for research and commercialization, particularly for small and medium-sized enterprises (SMEs).
Wide Range of Applications: Falcon's capabilities extend across various domains, including chatbots, customer service operations, virtual assistants, language translation, content generation, and sentiment analysis. Its adaptability makes it a valuable asset for businesses looking to enhance their language processing capabilities.
Steep Learning Curve for Novice Users: While Falcon offers impressive capabilities, users who are new to advanced language models will likely face a learning curve. Fine-tuning and customization may require some familiarity with the Hugging Face ecosystem and related tools.
Resource Intensive for Training: While the pre-trained models are readily available, training custom models from scratch may be resource-intensive, particularly for larger models like Falcon-40B. This could pose a challenge for individuals or organizations with limited computational resources.
Potential Overfitting in Fine-Tuning: As with any advanced language model, there is a risk of overfitting when fine-tuning Falcon for specific tasks. Careful consideration and validation of the fine-tuning process are essential to ensure optimal performance.
Continuous Model Updates and Maintenance: Staying up-to-date with the latest model versions and updates may require proactive monitoring and maintenance efforts. This is important to ensure that Falcon continues to perform optimally as the field of natural language processing evolves.
Our evaluation of Falcon LLM was conducted with meticulous attention to detail. We employed a structured approach, focusing on key criteria to ascertain its capabilities. Here are the four main criteria we used for testing Falcon:
Speed of Processing: We assessed Falcon's processing speed across various tasks, including text summarization and language translation. This criterion was crucial in determining its efficiency in real-time applications.
Ease of Use: We evaluated the user interface and documentation provided with Falcon. The ease with which users can navigate and utilize the model played a significant role in our assessment.
Accuracy in Language Tasks: Falcon's performance in language-related tasks, such as sentiment analysis and content generation, was rigorously tested. We compared its results to established benchmarks to gauge its accuracy.
Resource Utilization: We monitored the GPU memory usage and computational resources required to run Falcon. This criterion was essential in understanding the model's scalability and its suitability for different hardware configurations.
We employ a 5-star rating system for all the AI tools we review to give you a comprehensive idea of the overall utility of each tool.
Our team of experts has awarded this AI tool an overall rating of four stars. Falcon LLM, developed by the Technology Innovation Institute, has truly pushed the boundaries of language processing capabilities. Its innovative features like multi-query attention and flash attention, along with the availability of Falcon-40B and Falcon-7B variations, make it a versatile tool for various applications. The ease of fine-tuning and adaptability using the Hugging Face ecosystem adds to its appeal.
However, the learning curve for novice users and potential resource-intensive training for custom models are factors to consider. Overall, Falcon stands as an excellent choice for businesses and researchers seeking a powerful language model for diverse language processing tasks.
While the Falcon LLM, developed by the Technology Innovation Institute, offers impressive capabilities, it's essential to explore alternative options to ensure that you choose the best fit for your specific needs. Here, we introduce four noteworthy alternatives to Falcon, each with its unique features and advantages:
Orca is an advanced language model developed by Microsoft and is based on the LLaMA framework. What sets Orca apart is its fine-tuning of complex explanation traces obtained from GPT-4. This unique approach allows Orca to outperform many models, including Vicuna, on complex tasks. However, it's important to note that Orca is primarily designed for non-commercial use.
Pros:
- Exceptional performance on complex tasks.
- Fine-tuning GPT-4's explanation traces for improved accuracy.
Cons:
- Limited to non-commercial use, which will not be suitable for many business applications.
An open-source language model, BLOOM was developed as part of the BigScience Workshop, a collaborative effort involving Hugging Face and other research organizations. BLOOM was initially proposed as an alternative to GPT-3 and has since been superseded by models based on Meta's LLaMA.
Pros:
- Open-source nature allows for transparency and customization.
- Developed through a collaborative effort involving multiple research organizations.
Cons:
- May not offer the same level of performance as the latest commercial models like Falcon.
Cerebras-GPT is a series of language models developed by Cerebras, an AI accelerator company. These models were designed as a demonstration of Cerebras' Wafter-Scale Cluster technology. The models in this family are known for their scalability and efficiency in handling large-scale language processing tasks.
Pros:
- Scalability and efficiency make them suitable for large-scale applications.
- Developed by Cerebras, a company specializing in AI acceleration.
Cons:
- Limited information available compared to well-established models like Falcon.
An open-source library developed and maintained by LMSYS, FastChat is designed specifically for training, serving, and evaluating language models used in chatbot applications. It includes training and evaluation code, a model serving system, a user-friendly Web GUI, and a fine-tuning pipeline. It's the go-to system for Vicuna and FastChat-T5.
Pros:
- Specialized for chatbot applications, making it a powerful tool for conversational AI.
- Includes benchmarking features, ensuring performance optimization.
Cons:
- May have limitations when applied to non-chatbot tasks.
In summary, Falcon is undoubtedly a powerful and versatile language model with its unique features and advantages. However, the alternatives mentioned above also have their merits and cater to specific needs. When considering which model to choose, it's essential to assess your project's requirements, resources, and long-term goals. Ultimately, the choice between Falcon and its alternatives will depend on the specific demands of your project, your budget, and whether you prioritize open-source solutions or commercial models.
Check out our Universal Data Generator Review here.